Published by Bindi M. Doshi, PhD on Jul 15, 2024 9:38:00 AM
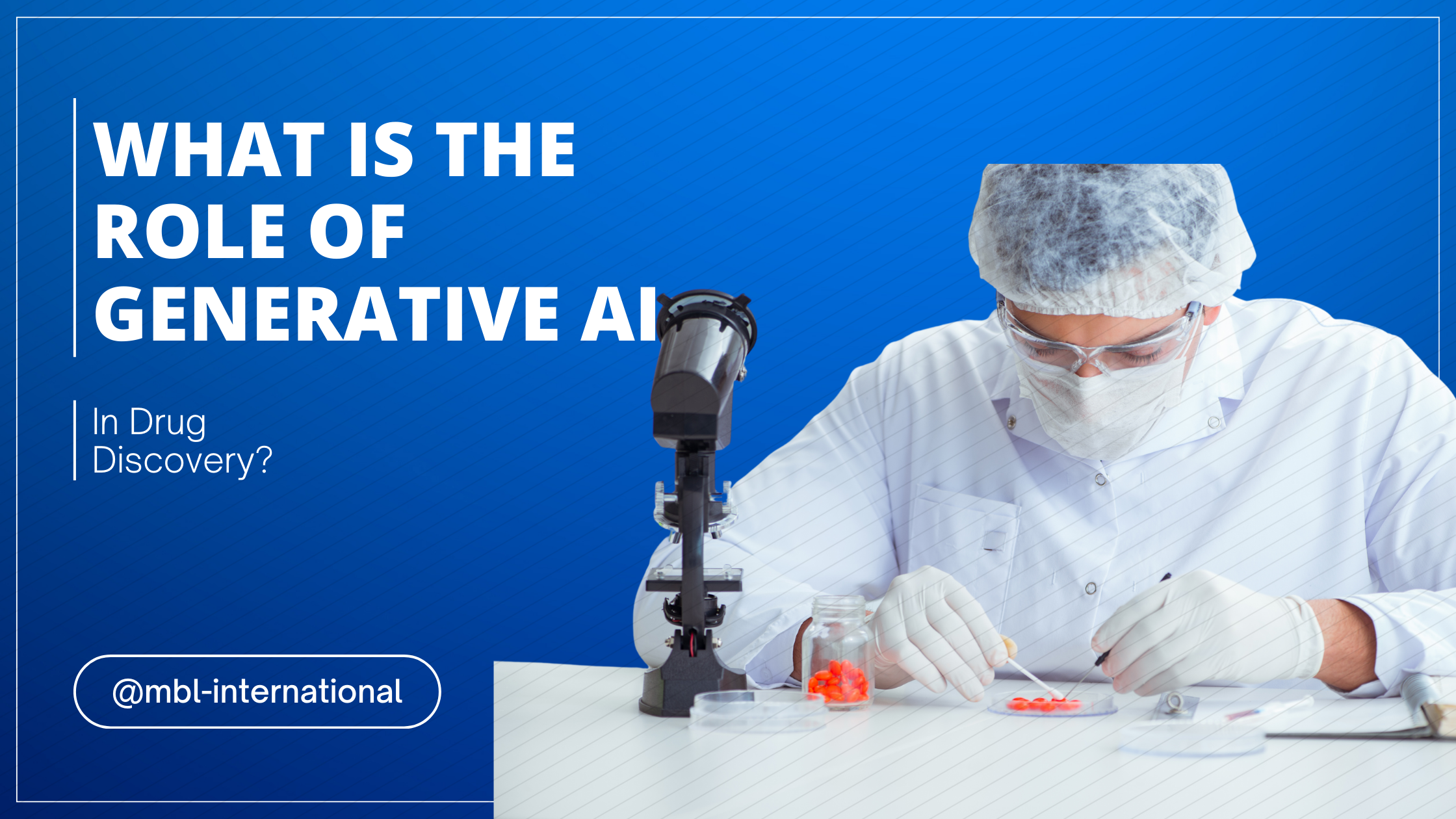
The integration of generative AI in drug discovery has emerged as a groundbreaking advancement in the pharmaceutical industry.
As we delve into the intricacies of this innovation, we must first understand the foundational question: what is drug discovery?
Drug discovery is the process by which new candidate medications are discovered.
Historically, this process has been extended, costly, and fraught with a high rate of failure.
However, the advent of generative AI promises to revolutionize this landscape by enhancing efficiency, reducing costs, and increasing the success rates of developing new drugs.
Understanding Drug Discovery
To appreciate the impact of generative AI, it is crucial to comprehend what drug discovery is in its traditional sense.
The drug discovery process typically involves several stages:
- Target Identification and Validation: Identifying biological targets (often proteins) that play a crucial role in disease.
- Hit Identification: Screening vast libraries of compounds to find molecules that can interact with the target.
- Lead Optimization: Refining these molecules to enhance their efficacy, reduce toxicity, and improve pharmacokinetic properties.
- Preclinical Testing: Evaluating the optimized compounds in vitro (cell cultures) and in vivo (animal models) for safety and efficacy.
- Clinical Trials: Conducting multi-phase trials in humans to confirm safety and efficacy.
- Regulatory Approval: Seeking approval from regulatory bodies to bring the drug to market.
Each of these steps is complex, time-consuming, and expensive.
The average cost of bringing a new drug to market can exceed $2 billion, and the process can take over a decade.
The Advent of Generative AI in Drug Discovery
Generative AI and intense learning models like Generative Adversarial Networks (GANs) and Variational Autoencoders (VAEs) have shown tremendous potential in transforming drug discovery.
These AI models can generate new data points from learned patterns in existing data, making them invaluable in identifying novel drug candidates.
Target Identification and Validation
In the initial stage of drug discovery, understanding what drug discovery is becomes essential when considering the role of generative AI in target identification and validation.
AI algorithms can analyze vast amounts of biological data, including genomic, proteomic, and transcriptomic data, to identify potential drug targets.
By learning patterns associated with disease mechanisms, generative AI can predict new targets with high accuracy, speeding up the target identification process.
Hit Identification
Traditionally, hit identification involves high-throughput screening of large compound libraries, a process that is both costly and labor-intensive.
Generative AI can simulate this process more efficiently.
AI models can generate virtual libraries of compounds with desired properties, significantly reducing the number of compounds that need to be physically screened.
This approach not only saves time and resources but also increases the likelihood of identifying promising hits.
Lead Optimization
Lead optimization is another area where generative AI excels.
Understanding what is drug discovery in this context involves recognizing the iterative nature of optimizing a drug candidate's properties.
Generative AI can predict how modifications to a molecule's structure will impact its biological activity and other pharmacological properties.
AI can guide chemists in designing more effective and safer drugs by generating new analogs of a lead compound.
Preclinical Testing
In preclinical testing, generative AI can predict a compound's behavior in biological systems.
AI models can simulate how a drug will interact with various proteins, cells, and organs, predicting potential side effects and toxicities.
This predictive capability allows researchers to prioritize the most promising compounds for further development, potentially reducing the number of animal studies required.
Clinical Trials
The application of generative AI extends into the clinical trial phase as well.
AI can analyze patient data to identify suitable candidates for clinical trials, design more efficient trial protocols, and predict outcomes.
This can lead to more targeted and effective clinical trials, increasing the likelihood of success and reducing the time and cost involved.
Benefits of Generative AI in Drug Discovery
The incorporation of generative AI into the drug discovery process offers numerous benefits:
- Speed: AI can analyze data and generate predictions much faster than traditional methods, significantly reducing the time required for drug discovery.
- Cost-Effectiveness: AI can reduce the overall cost of developing new drugs by streamlining various stages of the process.
- Innovation: Generative AI can identify novel drug candidates and new biological targets that traditional methods might overlook.
- Precision: AI models can provide highly accurate predictions, leading to better-targeted therapies with fewer side effects.
- Resource Efficiency: AI reduces the need for extensive physical screening and testing, conserving valuable resources and reducing waste.
Challenges and Considerations
While the potential of generative AI in drug discovery is immense, there are several challenges and considerations to address:
- Data Quality and Quantity: AI models require large amounts of high-quality data to learn effectively. Ensuring the availability and accuracy of such data is crucial.
- Interpretability: AI models, intense learning models, can be complex and challenging to interpret. Understanding the rationale behind AI-generated predictions is essential for regulatory approval and scientific validation.
- Integration with Existing Workflows: Integrating AI into traditional drug discovery workflows requires careful planning and collaboration between computational scientists and experimental biologists.
- Regulatory Hurdles: Regulatory bodies need to adapt to the use of AI in drug discovery, developing guidelines and standards for AI-generated drug candidates.
- Ethical and Societal Implications: The use of AI in drug discovery raises ethical questions regarding data privacy, bias in AI models, and the accessibility of AI-developed therapies.
The Future of Drug Discovery with Generative AI
The future of drug discovery is undeniably intertwined with the continued advancement of generative AI technologies.
As AI models become more sophisticated and capable of handling increasingly complex biological data, their impact on drug discovery will grow.
Personalized Medicine
One exciting frontier is the potential for personalized medicine.
Understanding what is drug discovery in the context of personalized medicine involves developing drugs tailored to individual patients based on their genetic, proteomic, and metabolic profiles.
Generative AI can predict how different patients will respond to various treatments, paving the way for highly personalized therapeutic approaches.
Accelerated Innovation
Generative AI will continue to accelerate innovation in drug discovery, enabling researchers to explore new chemical spaces and biological targets.
This will lead to the discovery of novel drug candidates that can address unmet medical needs and tackle complex diseases that have eluded traditional drug discovery methods.
Collaboration and Integration
The successful integration of generative AI in drug discovery will require continued collaboration between AI experts, biologists, chemists, and regulatory bodies.
By fostering interdisciplinary partnerships, the pharmaceutical industry can fully harness the potential of AI to revolutionize drug discovery and improve global health outcomes.
Conclusion
In conclusion, the role of generative AI in drug discovery is poised to transform the pharmaceutical industry.
By understanding what drug discovery is and recognizing the stages where AI can have the most significant impact, we can appreciate the profound changes AI brings to this field.
From target identification to clinical trials, generative AI enhances efficiency, reduces costs, and increases the likelihood of developing successful new therapies.
As technology continues to evolve, the integration of generative AI into drug discovery will lead to innovative treatments, personalized medicine, and improved health outcomes for patients worldwide.
To learn more about the transformative role of generative AI in drug discovery and its impact on the pharmaceutical industry, visit us at MBL International.
FAQs
What is drug discovery?
Drug discovery is the process of identifying new candidate medications. It involves several stages, including target identification, hit identification, lead optimization, preclinical testing, and clinical trials.
How does generative AI contribute to drug discovery?
Generative AI contributes to drug discovery by analyzing vast amounts of biological data to identify potential drug targets, generating virtual libraries of compounds for hit identification, predicting the effects of structural modifications during lead optimization, and simulating compound behavior during preclinical testing.
What are the main benefits of using generative AI in drug discovery?
The main benefits include faster data analysis, cost-effectiveness, the ability to identify novel drug candidates, increased precision in targeting therapies, and more efficient resource use.
Can generative AI replace traditional drug discovery methods?
Generative AI is not meant to replace traditional methods but to complement and enhance them. It streamlines various stages of the process, making drug discovery more efficient and effective.
What types of AI models are used in drug discovery?
Common AI models used in drug discovery include Generative Adversarial Networks (GANs) and Variational Autoencoders (VAEs), which can generate new data points from learned patterns.
What challenges are associated with using generative AI in drug discovery?
Challenges include:
- It is ensuring high-quality data for AI models.
- Interpreting complex AI predictions.
- Integrating AI into traditional workflows.
- Meeting regulatory requirements.
- Addressing ethical considerations.
How does generative AI help in personalized medicine?
Generative AI helps in personalized medicine by predicting how different patients will respond to various treatments based on their genetic, proteomic, and metabolic profiles, leading to highly tailored therapeutic approaches.
What is the role of generative AI in clinical trials?
In clinical trials, generative AI can analyze patient data to identify suitable candidates, design more efficient trial protocols, and predict trial outcomes, thereby improving the success rate and reducing time and costs.
Are there any successful case studies of generative AI in drug discovery?
Yes, companies like Insilico Medicine, Atomwise, and BenevolentAI have successfully used generative AI to identify novel drug candidates and potential treatments for diseases such as fibrosis, Ebola, multiple sclerosis, and COVID-19.
What is the future of drug discovery with generative AI?
The future of drug discovery with generative AI involves continuing to advance AI technologies, accelerating innovation, personalized medicine, and interdisciplinary collaboration to fully harness AI's potential in improving global health outcomes.